by Kathleen Fox
GOAL:
The goal of landscape grief is an exploration of the language we use to mourn online through the lens of Gertrude Stein. Gertrude Stein, when writing her plays, did not focus on the plot as the leading force in her story. She instead focused on the idea of landscape and used language to create landscapes that the reader could experience. The plot wasn’t the driving force, the language was. She used repetition and metaphor to create feeling. I wanted to an AI that could explore the language of grief in the way that Gertrude Stein writes her plays in.
DESCRIPTION
“Landscape Grief” is an exploration of the grief, how people publicly grieve on social media, and the writing style of Gertrude Stein. Gertrude Stein liked to write her plays as landscapes. Rather than having a clear narrative arc, Stein preferred to create landscapes of words. She used repetition and other poetic techniques to construct these landscapes that take the forms of play. The goal of “Landscape Grief” is to create an AI that can metaphorically paint a Gertrude Stein style landscape of what grieving looked like on Facebook in the wake of my father’s death.
My father passed away four years and he was a person who was thoroughly engaged with social media. When he passed away there was a whole world of grieving that only took place only on Facebook. People who I never knew were writing paragraphs upon paragraphs on my father’s Facebook page publicly grieving his death. It was truly bizarre. People whom I had never spoken to in my life, people who didn’t attend the funeral or people who frankly didn’t know my father outside of Facebook were creating this giant display of sorrow for months and years following his death.
I just think that the way we interact with social media and grief is so truly, deeply bizarre. There are no rules and the things people say can leave your jaw hanging down the side of your mouth – be it your college professors happily stating that she is so glad that her father is still alive at the age of 81 on a birthday post you made honoring your dad, or your dad’s childhood friend recounting a not at all appropriate for Facebook spring-break story for our entire family to see.
The goal of “Landscape Grief” is to create an AI that can process all these posts and comments in an attempt to try to understand what this kind of grief looks like. What is the language we use on social media to express grief? What does it look like and how does it sound?
I then crafted a video using the language the AI-generated combined with FB photos from my dad’s timeline.
PURPOSE AND INTENTIONS:
The purpose of the project was to create an AI that plays with the language of Social Media Grief. When my father passed away, a lot of grieving happened online. And while people no longer mourn him as they did four years ago, they will still post on his timeline.
It was something that made me very uncomfortable. The display feels performative and often inappropriate.
I’m in no position to approve of how people choose to grieve. There was a time in my life when I desperately wanted to delete my dad’s Facebook so I didn’t have to constantly have its presence on the edge of my mind.
The funeral ended. The posts never did. People still post all the time. I’m sure when June 14th rolls around (his death day) – a whole new load of posts will clog his timeline.
I let go of this desire to take my dad’s Facebook down but I was never able to pinpoint exactly why it made me so upset. Perhaps it’s because I couldn’t stand his physical death and digital life coexisting. Perhaps it’s because the mourning online felt insanely artificial.
I’ve always wanted to do something with my father’s timeline and this class felt like an excellent opportunity.
People are finding bizarre ways to grieve with the help of artificial intelligence. Creating AI’s of their loved ones like Luka’s Roman Mazurenko application.
I wanted to see explore the artificiality and the truth of the mourning by using Gertrude Stein.
Gertrude Stein is a master of repetition to create landscapes. Take a look at her excerpt from If I Told Him, A Completed Portrait of Picasso:
”If I told him would he like it. Would he like it if I told him. Would he like it would Napoleon would Napoleon would would he like it.
If Napoleon if I told him if I told him if Napoleon. Would he like it if I told him if I told him if Napoleon. Would he like it if Napoleon if Napoleon if I told him. If I told him if Napoleon if Napoleon if I told him. If I told him would he like it would he like it if I told him.
Now.
Not now.
And now.
Now.”
Similarly, the posts become repetitive on my father’s timeline. There are at least 100 variations or exact reputations of the following
“Happy Birthday in heaven”
“He was a great man, May he rest in peace”
“I’m so sorry for your loss”
Maybe the repetition isn’t just an artificial gesture but perhaps, just like Stein, the language is creating its landscape.
I fed the post and Stein’s work through GPT-2 to examine what is perhaps both artificial and true and to see the landscapes the repetition can create.
CULTURE:
We are looking through the lens of poetry, the western canon, the English language, and most importantly, the language of grief and digital mourning.
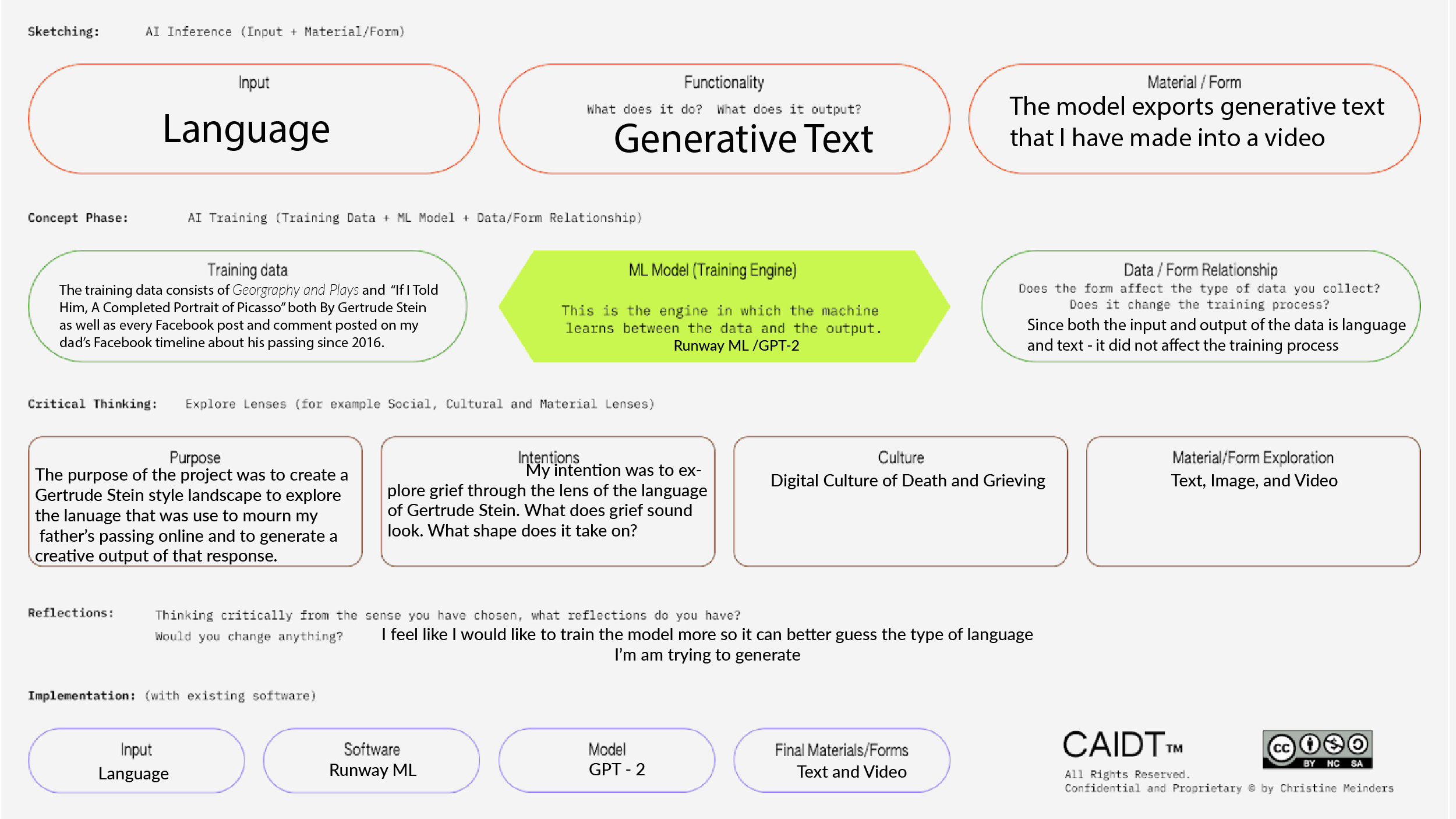
MATERIAL FORM / EXPLORATION:
While the text that the AI produced is very interesting on its own. I also wanted to explore the language in a more visual way. When my father passed away, I and others filled his timeline with photos and videos. I made a special memorial video. This too felt like a part of the grieving process online but there were a lot fewer photos and videos in comparison to texts. I manipulated the images and videos into a singular video. I then placed the texts on top of it so you can only see the realm of the video through the text. And because this entire Facebook Grieving posts feel like a funeral in their own right, I edited a funeral song and placed it in the background of the video.
INPUT:
The input the model worked with to generate text were the beginnings of texts from my dad’s Facebook posts like:
Your father
I’m so sorry
Marty was
I knew him
I can’t believe
And so forth and so on…
FUNCTIONALITY: (WHAT DOES IT DO? WHAT DOES IT OUTPUT?)
The model would output text in a generative fashion, drawing from the data I fed it. It would generate anywhere from 1 to 1024 characters. Some looked like this:
Input:
I can’t
Output:
I can’t imagine the pain
I can’t imagine the suffering
I can’t imagine the fire
I can’t imagine the dying
I can’t imagine the partying fool
I can’t imagine the partying fool
I can’t imagine the partying fool
Oh no I won’t mention it again
I won’t mention it again
In my mind’s eye
I won’t mention it again
In my mind’s eye
And in my fucking eye
(What I did) I didn’t do it again
I won’t mention it again
In my mind’s eye
And in my fucking eye
(What I did)
Input:
I’m so sorry
Output:
I’m so sorry. I’m so very sorry. I love you and miss you so much. May he rest in peace. amen
MATERIALS / FORM:
What the AI would output was text. Here is all the text that the AI-generated.
This along with images and videos from my father’s Facebook feed, an eerie digitized rendition of “On Eagle’s Wings” led to the final product.
Here is a link to the Runway Model
TRAINING DATA:
The GPT-2 model was trained on:
If I Told Him, A Completed Portrait of Picasso by Gertrude Stein
Geography and Plays by Gertrude Stein
My Dad’s Facebook Posts from June 14th, 2016 – Present
All the data is available here to view. The model was trained by paragraph to separate information. All the data has been combined in one document.
ABOUT GPT-2: (FROM RUNWAY)
Natural language processing tasks, such as question answering, machine translation, reading comprehension, and summarization, are typically approached with supervised learning on task-specific datasets. We demonstrate that language models begin to learn these tasks without any explicit supervision when trained on a new dataset of millions of webpages called WebText. When conditioned on a document plus questions, the answers generated by the language model reach 55 F1 on the CoQA dataset – matching or exceeding the performance of 3 out of 4 baseline systems without using the 127,000+ training examples. The capacity of the language model is essential to the success of zero-shot task transfer and increasing it improves performance in a log-linear fashion across tasks. Our largest model, GPT-2, is a 1.5B parameter Transformer that achieves the state of the art results on 7 out of 8 tested language modeling datasets in a zero-shot setting but still under its WebText. Samples from the model reflect these improvements and contain coherent paragraphs of text. These findings suggest a promising path towards building language processing systems which learn to perform tasks from their naturally occurring demonstrations
ACKNOWLEDGMENTS:
This project was created as a part of the AI. Culture. Creativity. Class at CalArts. Thank you to Christine Meinders for the constant support and guidance.